The high performance of modern research infrastructures poses two main problems for the analysis of spectra and similar measurement data: On the one hand, many measurements achieve good statistics after a short time, though the labor-intensive and time-consuming evaluation by highly qualified personnel remains as a bottleneck. For an automated evaluation, classical approaches are only suitable to a limited extent due to the high dimensionality of the parameter spaces to be analyzed.
On the other hand, the high sensitivity makes it possible to detect even the smallest signals—but with such noisy measured values that conventional analysis methods have difficulty determining them. However, these problems are countered by an enormous amount of already evaluated spectral data from a wide variety of measurement techniques, which lend themselves as training data for data-driven evaluation methods. In this project, Machine learning (ML) methods will be used to mitigate the complexity of the large parameter spaces. The status quo in this area is a patchwork of different solutions, which are specially which are specifically adapted to the respective applications and are therefore difficult to extend.
The main objective of the project is therefore to provide a generic ML approach for the automated evaluation of spectral datasets of different physical measurement methods – such as phase analysis with X-ray or neutron diffraction, neutron spectroscopy, elemental analysis by nuclear radiation or astrospectroscopy – on the basis of physically interpretable models. The approach is deliberately applied in parallel to several techniques to ensure that it is sufficiently general and can be extended to other measurement methods at a later stage.
Project duration: 01.03.2023 – 01.03.2026
This project is funded within the action plan ErUM Data of the German Ministry for Education and Research (BMBF).
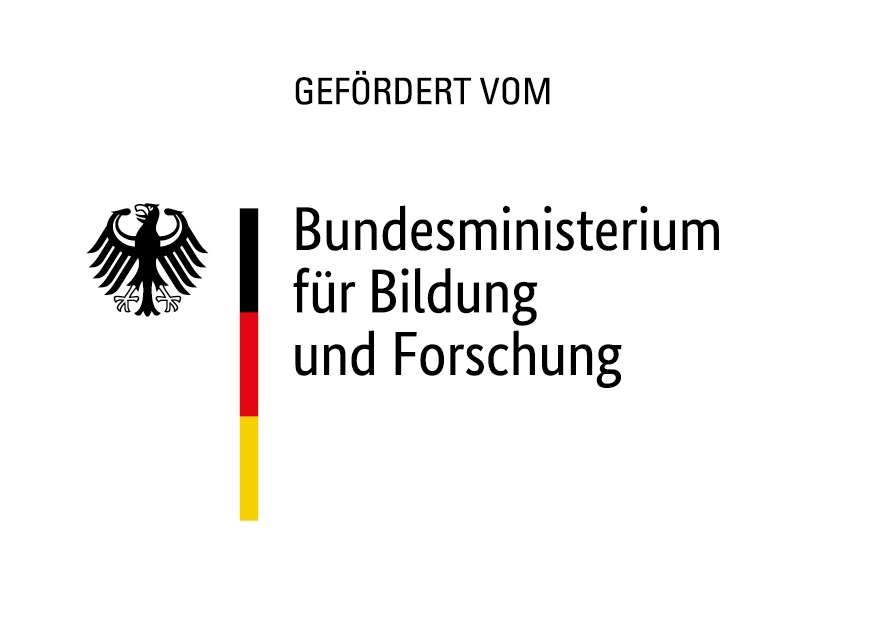
Letzte Änderung: 20. November 2023