The following graphic shows the content-related, methodological and technical competencies at the institute.
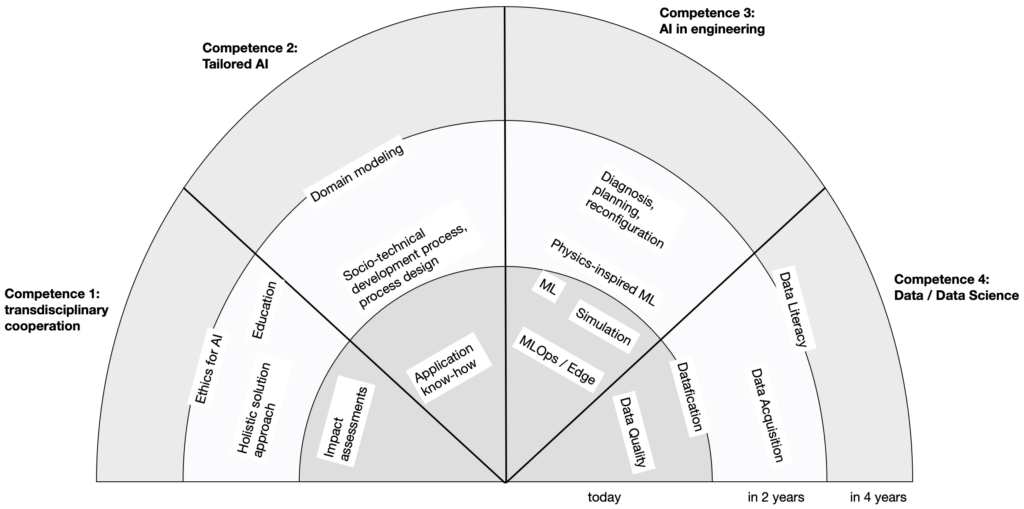
Skill 1: Transdisciplinary cooperation
In order to be able to develop and reflect on holistic approaches to solutions in the field of AI, transdisciplinary perspectives are increasingly indispensable. For example, questions of ethics or the social compatibility of technology solutions require an intensive exchange between different disciplines, which systematically includes critical reflection on their own concepts or value assumptions. This reflective view must also be the basis of corresponding educational approaches.
Skill 2: Tailored AI
Depending on the specific application, the data situation, requirements or demands on behalf of the human part in the interaction between humans and machines (AI) or the social implications, different types of AI become relevant. This refers, for example, to the spectrum of directly interpretable, so-called white-box models on the one hand and pure black-box methods on the other, and in this context often requires an application-dependent balancing of interpretability and prediction quality. In the field of prescriptive decision analytics, the range of methods ranges from methods for deterministic optimization problems, to multi-level sequential decision problems under uncertainty, to decentralized coordination problems with autonomous actors, depending on the application requirements. In addition, the quality and amount of available data and variables can vary greatly in different applications. So it will hardly be possible to do justice to every application and every situation with a single type of AI system. In this context, HSU-AI offers a holistic view of questions of process design, which includes variables of very different types and dimensions.
Skill 3: AI in Engineering
The results of non-technical ML applications are usually interpreted and verified by a human. Using ML in a technical system, on the other hand, often means that the application is done in a closed loop: the results are interpreted by software and then automatically used for optimization. This different usage scenario creates challenges that differentiate CPS from non-technical ML applications. At its core, higher robustness and resilience are required of AI systems in engineering: (i) The main characteristic of all physical systems is that their behavior must be considered over time and not just at a specific point in time – therefore, for example, the ML methods used must also take into account and predict system behavior over time. (ii) In order to be able to use ML methods and learned models in CPS, it is imperative to evaluate the uncertainty of the predictions of the ML systems. Uncertainties often arise from noise in the sensor data, measurement errors or from unobservable values or disturbances (“confounding effects”). (iii) For CPS, there is already extensive prior knowledge in the design phase, which is based on physical laws and technical knowledge. This individual knowledge is to be used to improve AI practices. Especially with ML solutions, prior knowledge can reduce the need for large amounts of data. (iv) AI results and generated models need to be explained to human operators: this requires learning symbolic concepts from the (numerical) models. Explanations and justifications are generated for this purpose.
Skill 4: Datafication and Data Literacy
In the sense of the approaches described, HSU-AI is characterized by a multi-perspective approach to the topic of data and data science. For example, data literacy models play a major role in understanding and reflecting on the design of AI systems. Likewise, the term datafication helps to grasp the comprehensive socio-technical structural contexts of AI and make them editable. Semantic technologies help improve data quality and the quality of AI systems by enabling a deeper understanding of the content of data and its context. In addition, careful data allocation is crucial to ensure that AI algorithms are trained with high-quality and representative data. AI approaches have always been used in the cognitive sciences in the modeling of mental processes.
Letzte Änderung: 27. June 2024